Australia has famously enjoyed almost 30 years without an economic recession, and Vivek Pradhan thinks that presents a unique data challenge for the nation's banks.
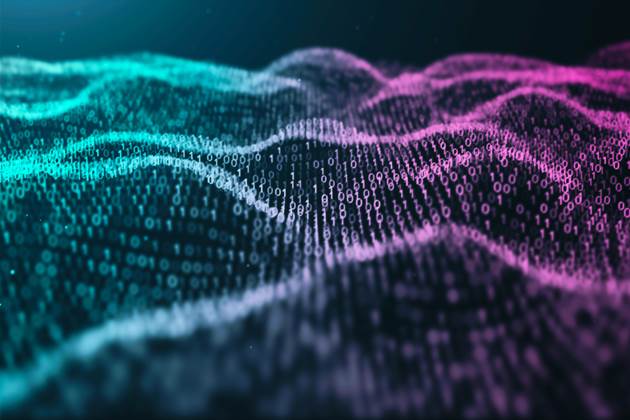
Pradhan is a Partner at KPMG who specialises in helping organisations to unlock new value from large-scale data platforms, and building digital solutions. His work contributes to KPMG’s Digital Delta team, which focuses on digital transformation.
To outline his theory of a strong economy being challenging for banks, Pradhan explains that “One of the things a retail bank does is build and train credit risk models to ensure they reserve enough capital to allow for bad debt. To do that they have risk models that look at historical data around delinquency and defaulting on credit cards and other data to help them make that decision.”
But with Australia’s last recession ending in 1991, Pradhan worries that “Banks’ ability to build risk models that are stress tested is limited to a certain extent, because the dataset is not representative.” There just aren’t enough lean years in their records to help them predict the impact of a downturn.
Yet banks have it relatively easy because they naturally collect a lot of data about their customers’ finances. Pradhan therefore thinks organisations face bigger obstacles to gathering trustworthy data and putting it to work deriving actionable insights.
“I think there's still a huge challenge for most large organisations to create the necessary capability for a data-driven culture,” he says.
One aspect of that challenge is ensuring workers become more “data-literate” and learn data interpretation skills, rather than concentrate them in IT departments or specialist pockets.
“This is not the skill set of a statistician or a data scientist,” Pradhan says. “It’s more about using the data available to tell a story from a set of data points over a period of time. It’s not about having great math skills.”
Being willing to set aside other skills can also be important.
Pradhan relates the story of an organisation that wished to improve its sales and marketing function, but at which senior management had built careers on decisions made largely by instincts honed over many years of hands-on work on the factory floor. “It's hard to transform that mindset to look at how specific individuals make decisions,” he says.
If an organisation can get this right, he thinks they can reap the benefit of automating routine tasks and freeing people to do more abstract and important work that requires human skills.
But none of the above changes are possible, or can deliver impactful results, without good data.
“You must have a trusted, curated dataset, able to feed that into training the models, and be confident that that those the training data sets are representative of a real world data set,” he says.
And generating that’s as much an ethical challenge as it is an organisational concern.
“I think lots of organizations have the view that if you have a diverse cohort of people tasked with designing automation systems, you're less likely to put in intrinsic bias,” Pradhan says. “That’s true to a certain extent, but it takes a lot more than just a diverse workforce.”
Others have also raised concerns about ethics.
In early 2019 the Federal Department of Industry, Innovation and Science published a national AI ethics framework and accompanying AI ethics principles.
CSIRO Research Scientist Emma Schleiger and Data 61 Senior Principal Scientist, Strategy and Foresight, Stefan Hajkowicz, contributed to those documents’ development. Schleiger and Hajkowicz called for “particular attention to ensure the ‘training data’ is free from bias or characteristics which may cause the algorithm to behave unfairly.” Without that care, the pair worried about “unfair discrimination against individuals, communities or groups.”
“There is a pressing need to examine the effects that AI has on the vulnerable and on minority groups, making sure we protect these individuals and communities from bias, discrimination and exploitation,” the pair wrote.
Some commentary on the draft ethics framework pointed out that data-driven services sometimes circumvent established checks and balances.
Free TV Australia, which represents Australia’s free-to-air television broadcasters, pointed out (pdf) that its Commercial Television Industry Code of Practice requires broadcasters to “present factual information accurately” and that “news programs be presented fairly and impartially.”
But the organisation points out that Google and Facebook use AI and data-driven techniques to choose what news to present their readers, and have no obligation to be fair or impartial. Nor are social networks regulated.
Software giant Salesforce’s Senior Director of Government Affairs & Public Policy for APAC and Japan Sassoon Grigorian responded to the framework by suggesting the extra step of an “Australian national advisory council on ethics and AI” featuring “members representing a mix of sectors including business, not-for-profit, academic and government, and a diverse range of backgrounds that reflect community diversity. It should also not be limited to technologists, including human rights advocates, ethicists, economists and community members.” Grirgorian also suggested (pdf) that businesses need similar in-house councils.
National Australia Bank called (pdf) for AI at scale to be considered, because “the complexity of AI, including the ingesting of and learning from data, is often manageable during pilots and isolated use cases, however ‘becomes exponentially more difficult to address’ when AI systems interact and build on each other.”
The Bank, together with Microsoft, Telstra and the Commonwealth Bank, have since signed up to test the AI ethics principles.
KPMG’s Pradhan welcomes the ethics conversation, because he feels that trust is essential if data-driven decision making is to be accepted by Australian businesses and their customers. And today, he feels that more work is needed to build trust.
“There are a lot of AI experiments that haven't been operationalised,” he says. “It's one thing to have a data scientist to then build a model, it's entirely another to actually put that model into an operational context and have it drive decisions.”
“I don’t think that a lot of organisations are at that point yet where they're able to operationalize machine learning and automation capabilities.”
“The underlying technology and infrastructure that you need to operate are still developing,” he says. “I think it comes back to a combination of skills and but also having trust in the data, the decisions that come out and how to actually create trust.
“When we can do that, we’ll inspire trust too.”