A little information can be dangerous. That may be a well-worn cliché, but it is one business and technology leaders should keep in mind as they look to use artificial intelligence (AI).
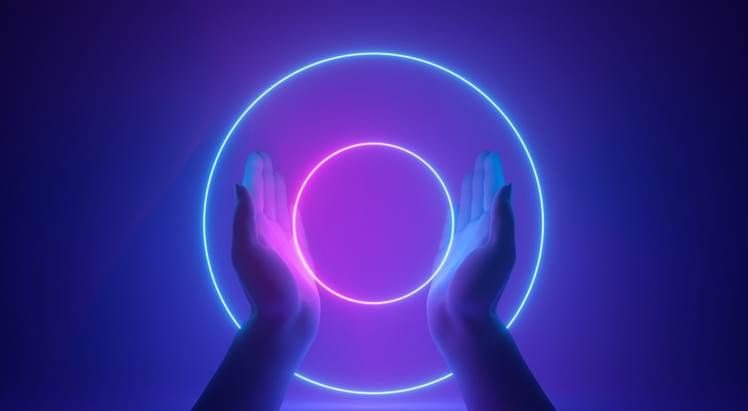
As use of AI increases, so does the risk that poorly trained AI systems will cause problems in business and government. Gartner once forecast that 85 per cent of AI deployments would deliver erroneous outcomes through 2022.
To avoid this, companies need large volumes of accurate data to train AI systems, and people who understand how the algorithms might interpret the data.
But that isn’t the entire picture when it comes to ensuring accurate and safe use of AI. Organisational leaders are also being encouraged to look beyond the data at how much power they give AI. They are being urged to use it to support, not replace, human decisions.
The need for human oversight of AI is well-known. But as more is learned about this, the implications for business and government are becoming clearer.
Shane O'Sullivan, an AI and cognitive expert within the Digital Delta team at KPMG Australia, cautions companies to see AI as a means to ‘augmented intelligence’ rather than as an independent decision maker.
Without people, AI is nothing, O’Sullivan points out. AI algorithms are good at “generally dealing with very large volumes of data and sorting through it in a way that humans just couldn’t,” he explains, “then synthesising that down into information that humans can then complete a decision around. The human counterpart is needed to deal with the more complex activities.”
“AI is helping people do things much better, much faster, and much more efficiently than they could have done before.”
The key word – helping – highlights the mistake underlying some AI implementations, where managers hand too much power to the technology.
“AI is still a bit mysterious to many people,” O’Sullivan says, “because it is a really broad church, ranging from image recognition to natural language processing, machine learning and many other capabilities. So the best conversations we have start with ‘I’ve got a problem’ – and then we work together towards solving that problem.”
AI “is actually really narrow in terms of what it actually gets trained to do,” O’Sullivan points out. A computer can’t identify a dog if all it has ever seen is photos of cats, so AI systems are only as good as the people teaching them and the data available for training, he comments.
“Generally, companies train AI to very specific and somewhat narrow use cases,” he explains, “and we are still many years away from generalised AI that can mimic what the brain does – so for the next decade or so, we describe ourselves as being in the era of augmented intelligence, in which humans and machines are working together.”
Play to our strengths
Management consultants have warned companies to prepare their workforces for layoffs as automation supplants jobs lost during the pandemic. Yet, the World Economic Forum has suggested that AI will create more jobs than it eliminates. It expects a surplus of new jobs by 2025 due to an increased need for specialised digital skills and the elimination of repetitive and manual jobs.
Deciding how those jobs will change – and which ones will change – should therefore be part and parcel with any AI rollout.
The key to augmenting workers successfully is to respect the boundary between AI’s capabilities and those of the humans relying on it, says Professor Toby Walsh, a UNSW School of Computer Science and Engineering researcher. Walsh’s work in AI with CSIRO’s Data61 division earned him an appointment as a Fellow of the American Association for the Advancement of Science.
“Humans are no good at working on probabilities, but computers are really good at it – and they can look at data sets larger than what humans can look at,” Walsh explains.
The success of AI-powered chess computers, he said, was not due to the creation of a sort of programmatic intelligence, but instead a result of their ability to evaluate and score massive sequences of possible moves in an instant.
“On the other side, machines don’t have our creativity, our adaptability, our social and emotional intelligence today – and it’s not clear if they ever will.”
These differences have implications for corporate AI strategies: “We should be playing to our strengths,” Walsh advises, “and not trying to compete against machines – because there’s plentiful evidence that whenever we’ve gotten a computer to do some task, it has soon eclipsed us and left us far behind.”
In industrial applications with specific scope, Walsh says, AI’s ability to manage dangerous real-world tasks has made it invaluable. This includes in industries such as mining, where AI-powered self-driving vehicles and trains have increased efficiency and driven down operational costs.
“We don’t employ that many fewer people in mining today,” Walsh says, “but people are employed doing nicer, safer, less dangerous things – and mining remains a significant part of our economy as a result of this.”
Punching above our weight
Lives may not be at stake in every application of AI, but business decisions and operations are on the line – especially in data-driven organisations.
The global ‘datasphere’ will expand from 45 zettabytes in 2019 to 175 zettabytes by 2025, according to one widely cited IDC estimate. It predicts that nearly 30 per cent of that data will require real-time processing.
AI’s role in processing that data ranges from the pedestrian – scanning data sets for errors and internal inconsistencies, for example – to high value-added applications such as anomaly detection, contract vetting, complex trend analysis and sophisticated forecasting.
For example, an Australian telecommunications provider sought to understand why the cost of customer support was exploding whenever there was an outage in the Telstra backbone or National Broadband Network.
An AI system was built to monitor normal behaviour on those networks, KPMG Australia telecommunications data and analytics leader Phil Thornley says. The system quickly identified changes in network performance and raised trouble tickets that guided human engineers to do more-sophisticated network diagnostics.
“You’ve got a higher level of automation in place, where proactive customer engagement and notification of a ticket needed to be solved,” Thornley explains. “This is a case where AI can handle this much today – and as more data is gathered and more models are trained, it will keep doing more and more.”
A staged approach
O’Sullivan recommends companies step through their augmented intelligence project in five stages – building the AI capability; establishing policies and guidelines for augmented intelligence around areas such as trust, bias and liability; building an operating model for human/machine teaming; investing in a culture of trust so humans fully leverage the machine contribution in an activity; and mapping both technical and human paths to increasing levels of automation.
This is in line with Gartner’s previous prediction that 20 per cent of companies would dedicate workers to monitoring and guiding the neural networks on which their future business state is founded.
“You’ve got to get the right decision points in place and you don’t code too much into the algorithm,” says Thornley, “so you have that balance of putting facts in front of the human – so they make the decision, rather than trying to put too many decisions in the AI.”